Hideki Yamaguchi
- yamaguchi.h.et.al [at] gmail.com
- hideki-yamaguchi.com
- Yokohama, Japan
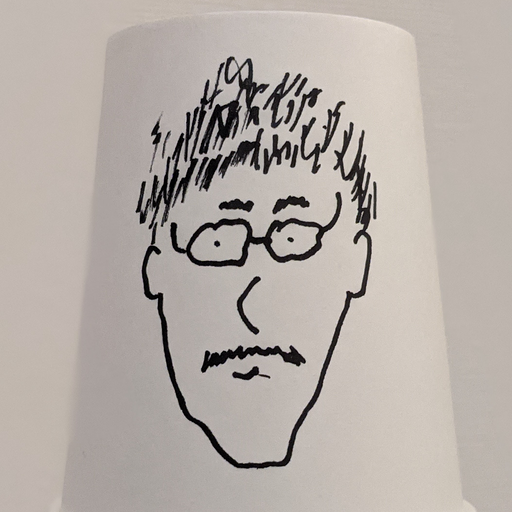
Hi, this is the web site of Hideki Yamaguchi. I am a data scientist in a pharma, trying to develop innovative machine learning methods for efficient molecular design. My academic background is theoretical statistical physics (M.Sc., statistical-physical analyses on biological macromolecules) and computational biology (Ph.D., language model-based protein design).
Interests: Machine learning, protein engineering, drug discovery, software development, problem solving.
Research achievements
Publications (peer-reviewed)
- Matsushita, T., Kishimoto, S., Hara, K., Hashimoto, H., Yamaguchi, H., Saito, Y. & Watanabe, K. (2024). Functional Enhancement of Flavin-Containing Monooxygenase through Machine Learning Methodology. ACS Catalysis, 14(9), 6945–6951. doi: https://doi.org/10.1021/acscatal.4c00826
- Yamaguchi, H. & Saito, Y. (2023). Protein language models. JSBi Bioinformatics Review, 4(1), 52-67. doi: https://doi.org/10.11234/jsbibr.2023.1
- Ogawa, Y., Saito, Y., Yamaguchi, H., Katsuyama, Y., & Ohnishi, Y. (2023). Engineering the Substrate Specificity of Toluene Degrading Enzyme XylM Using Biosensor XylS and Machine Learning. ACS synthetic biology, 12(2), 572–582. https://doi.org/10.1021/acssynbio.2c00577
- Yamaguchi, H. & Saito, Y. (2022). EvoOpt: an MSA-guided, fully unsupervised sequence optimization pipeline for protein design. Machine Learning for Structural Biology Workshop, NeurIPS 2022. [paper]
- Yamaguchi, H., & Saito, Y. (2021). Evotuning protocols for Transformer-based variant effect prediction on multi-domain proteins. Briefings in bioinformatics, 22(6), bbab234. https://doi.org/10.1093/bib/bbab234
Presentations (peer-reviewed)
- H.Y.. Protein design via natural language. ‘Eight problems in bioinformatics’ workshop in IIBMP2023 (keynote speaker).
- H.Y. and Yutaka Saito. MSA-guided, data-efficient sequence optimization algorithm for protein design. IIBMP2022 (poster).
- H.Y. and Yutaka Saito. Zero-shot property prediction and sequence exploration by protein language models. IIBMP2021 (poster).
- H.Y. and Yutaka Saito. Evotuning protocols for Transformer-based variant effect prediction on multi-domain proteins. IIBMP2021 (oral).
- Tomohiro Oniduka, H.Y., and Yutaka Saito. Development of multi-task learning method for optimizing properties of antibody drug. IIBMP2021 (poster).
- Shuzo Fukunaga, H.Y., and Yutaka Saito. Improvement in variant effect prediction accuracy with an augmentation by fitness translocation. IIBMP2021 (poster).
- H.Y. and Yutaka Saito. Evotuning protocols for Transformer-based variant effect prediction on multi-domain proteins. ISMB/ECCB2021 Special Session Representation Learning in Biology (Invited lightning talk). [Recorded presentation]
- H.Y. and Yutaka Saito. Accurate prediction of variant effects by efficient incorporation of evolutionary information into Transformer-based deep learning. PSSJ2021 (poster).
- H.Y. and Yutaka Saito. Evolutionary training protocols for deep representation learning of multi-domain proteins. IIBMP2020 (poster).
- Shuzo Fukunaga, H.Y., and Yutaka Saito. Correlation analysis between directed and natural evolution of proteins using deep representation learning. IIBMP2020 (poster).
Career
Head of machine learning group
As the manager of machine learning group in the research division, I lead the team of data scientists and research engineers to integrate really impactful ML technologies into therapeutic molecular design processes.
Data scientist
In the research division, I was involved in developing impactful machine learning methods for efficient molecular design.
Research engineer
SyntheticGestalt K.K. is an early-stage company based in Tokyo and London. As a research engineer, I developed cloud-based machine learning systems for drug discovery and industrially-useful enzyme design. Graph neural networks or large protein language models were my primary toolkits.
Ph.D. student
As a Ph.D. student in the Department of Computational Biology and Medical Sciences (CBMS), Graduate School of Frontier Sciences, I studied protein language models and its appliactions to efficient protein design. I was also a visiting student in the Artificial Intelligence Research Center, National Institute of Advanced Industrial Science and Technology (AIST). Supervised by Dr. Yutaka Saito. Doctoral thesis title: “Data-efficient protein design based on protein language models”.
Senior algorithm engineer
PKSHA Technology Inc. is a leading machine learning company in Japan, span out from a machine intelligence lab in the University of Tokyo. In the company, I developed multiple algorithms for computer vision, data analysis, or chemoinformatics, based on deep learning and other ML techniques. My clients were a number of top-tier companies in automobile, surveillance, healthcare, pharma, and other industries. Python was the main tool (mostly PyTorch for deep learning but sometimes TensorFlow v1/v2, OpenCV, and PyData stack).